2024: The Rise of Agentic AI in the Enterprise
The rise of agentic AI marks a pivotal shift in enterprise technology, offering unprecedented opportunities for automation, efficiency, and innovation. Defined as LLM-powered systems capable of reasoning and executing complex tasks without human intervention, AI agents are set to transform business operations across industries. While venture capital investment in agentic AI has surged, true impact and advancement will only come with widespread adoption at the enterprise level. However, with the technology in its early stages, we have yet to see meaningful uptake. To better understand the factors driving or hindering adoption—and to help founders developing AI agents for the enterprise accelerate this process—we surveyed 100 senior enterprise IT decision-makers across the U.S. and interviewed leading voices in AI innovation and investment.
Our findings reveal a disparity in readiness for AI adoption. Twenty-nine percent of enterprise leadership teams have a near-term vision (one to three years) to achieve enterprise-wide AI adoption, defined as AI being a critical part of at least five core functions. However, a larger portion—46%—anticipates achieving this level of adoption in the longer term (three or more years).

Nevertheless, interest in and preparation for AI agents is growing. Nearly half (48%) of enterprises are already adopting agentic solutions, with an additional 33% actively exploring solutions.

When deciding on AI solutions, a significant 63% of executives report adopting a hybrid approach—combining in-house AI models with third-party solutions. This strategy allows businesses to retain flexibility and control by developing custom solutions while leveraging the cutting-edge innovation of third-party providers. This measured approach reflects the careful balancing act that enterprises are navigating—harnessing AI's potential while mitigating the risks associated with this emerging technology.

As we dive deeper into the data, this report will explore the key challenges and opportunities facing enterprises in their journey to adopt AI agents. Taking insight from industry leaders, we'll address the barriers of trust, security, performance, and scalability and highlight proven strategies for startups to succeed in the space.
Contributors
- Sharon Zhang, Co-founder and CTO of Personal AI, a privacy-first platform enabling enterprises to create digital "twins" of employees to enhance productivity.
- Tooba Durraze, Ph.D., CEO of Amoeba, an AI-powered data scientist for revenue growth.
- Taylor Black, Director of AI & Venture Ecosystems, Office of the CTO at Microsoft.
- Sam Strickling, Senior Director of Growth and Innovation at Fortive, a global industrial technology company providing essential software, hardware, and services.
- David Magerman, Partner at Differential Ventures and Ph.D. in Computer Science and Quantitative Investing.
- Tim Guleri, Managing Partner at Sierra Ventures, a seed and Series A fund investing in AI enterprise solutions.
- Jonah Midanik, General Partner and COO at Forum Ventures, an early-stage B2B SaaS fund, accelerator, and AI venture studio.
Trust is Paramount to Adoption
Despite the transformative potential of agentic AI in automating and revolutionizing enterprise operations, trust emerged as the key obstacle preventing its widespread adoption across industries. Concerns over data accuracy and privacy, along with the overall performance of AI agents, are causing many decision-makers to take a more cautious approach.
Fifty-five percent of survey respondents identified one of the following trust concerns as a top barrier to adoption: data privacy (13%), performance and reliability (13%), accuracy, efficacy, and appropriateness (8%), ethical issues (5%), industry regulations and legal standards (4%), and too many unknowns (12%).
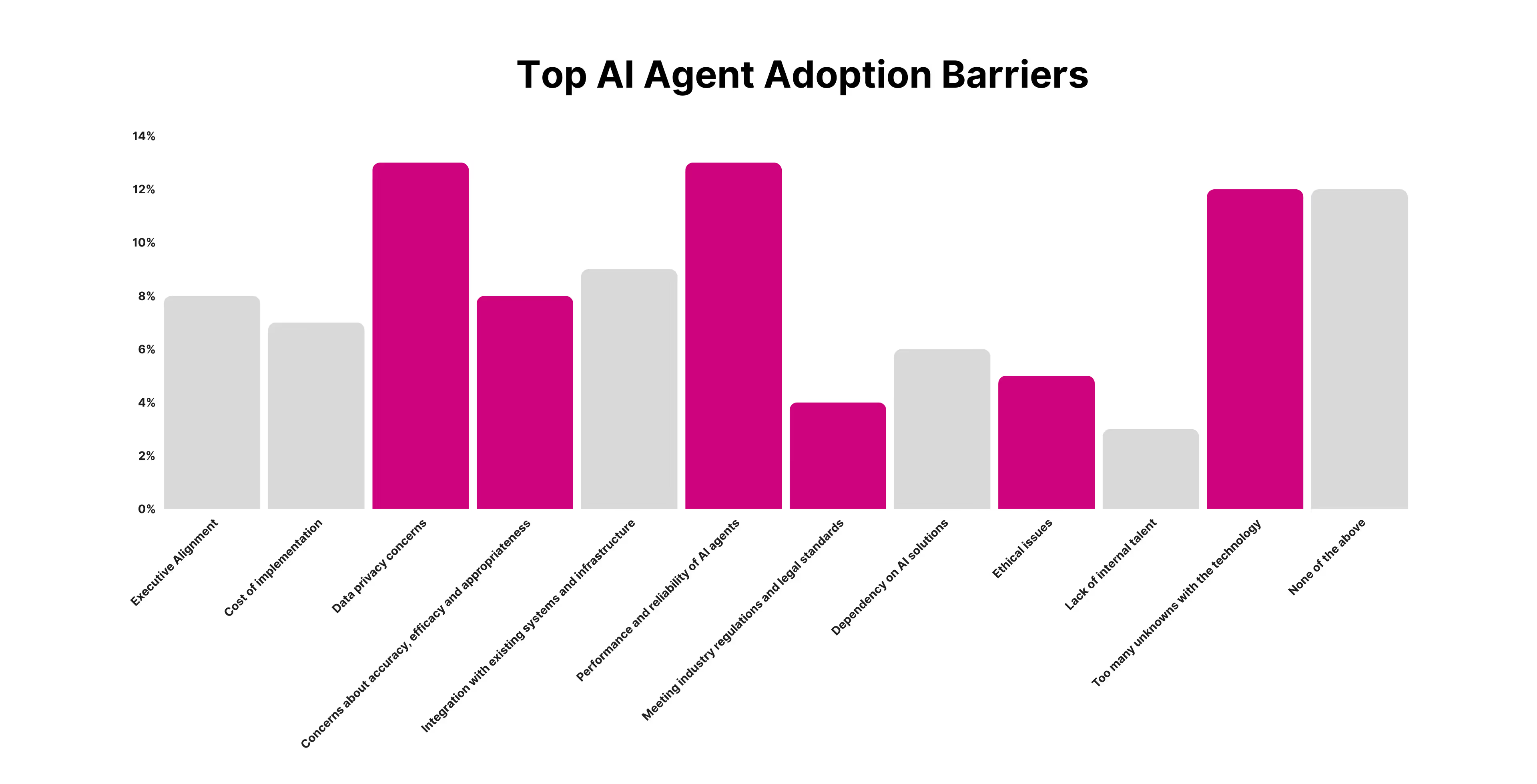
"The trust gap is enormous. While AI agents can perform tasks with remarkable efficiency, their outputs are based on statistical probabilities rather than inherent truths,” Jonah Midanik, General Partner and COO at Forum Ventures, explains. “This makes it crucial for startups to build systems that are not only accurate but also transparent and reliable.”
In September 2024, Anthropic released its enterprise solution, which focuses primarily on security. It features role-based access and permissioning, centralized provisioning control, audit logs for compliance monitoring, and training on internal knowledge. This affirms the imperative for privacy—and security-first solutions in the enterprise.
Tim Guleri, Managing Partner at Sierra Ventures, emphasizes, “Enterprises need confidence that their data remains secure, and the AI agents' outputs are aligned with company values and policies. Without these assurances, enterprises will hesitate to deploy AI agents, especially as they become more autonomous. Policies and frameworks to manage this trust gap will be key to accelerating adoption.”
Until AI agents can consistently demonstrate their value and mitigate risks, enterprises are likely to proceed cautiously, prioritizing smaller-scale implementations and pilot projects over full-scale adoption. The following outlines three critical approaches for building trust with enterprise customers to speed up adoption.
1. Prioritize Transparency
Enterprises want to see what is happening behind the curtain."They need to understand how AI agents make decisions, and they need to trust that these decisions are reliable," explains Guleri.
To tackle this, startups should provide clear documentation of decision-making processes. This can be achieved through explainable AI (XAI) frameworks that break down how an agent arrives at its conclusions in a way that is both technical and understandable to non-technical stakeholders. Startups should also offer audit trails that track decision points and offer visibility into how data flows through the system. Regularly updating these documents to reflect changes in the model or decision-making processes will further enhance trust.
Taylor Black, Director of AI & Venture Ecosystems at Microsoft, highlights that transparency is needed not only in how decisions are made but also in how data is used. Transparency is crucial for enterprises to feel confident that AI agents will act predictably and in line with organizational goals.
Sharon Zhang, founder of Personal AI, digital employee "twins" for the enterprise, has had success with this in highly regulated industries by keeping all user indexes separate, and isolating every data point and model. “This separation ensures users feel confident that their data is not being mixed with others or used to train broader models.”
2. Ensure Compliance and Security
Security and compliance are key drivers of adoption for enterprises, with 36% of survey respondents identifying them as the most significant factors influencing their decision to invest in AI Agent solutions.

In addition, 23% stated that if there was clear evidence that AI Agents can perform tasks more accurately, safely, and effectively, then their speed to adopt an AI Agent solution would be greater.

“Without strong security and compliance frameworks, enterprises will be hesitant to adopt AI solutions, regardless of their potential benefits," explains Midanik. “Startups must integrate robust data protection measures and demonstrate adherence to industry standards.”
Midanik advises startups to layer on a solution like Private AI, a de-identification system that enables the enterprise to comply with global privacy regulations like the GDPR, CPRA, HIPAA, or Pegasi, which corrects AI errors in real time. It can provide the necessary security assurances to accelerate enterprise adoption of AI agent solutions.
3. Build a Human-in-the-Loop Framework
User control and human oversight are critical components for the successful adoption of AI agents in enterprise settings. Twenty-two percent of respondents cited the need to maintain human control over AI decisions, particularly in critical applications, as their top ethical concern with AI agents. For highly regulated industries like legal and healthcare, human review will always be essential.
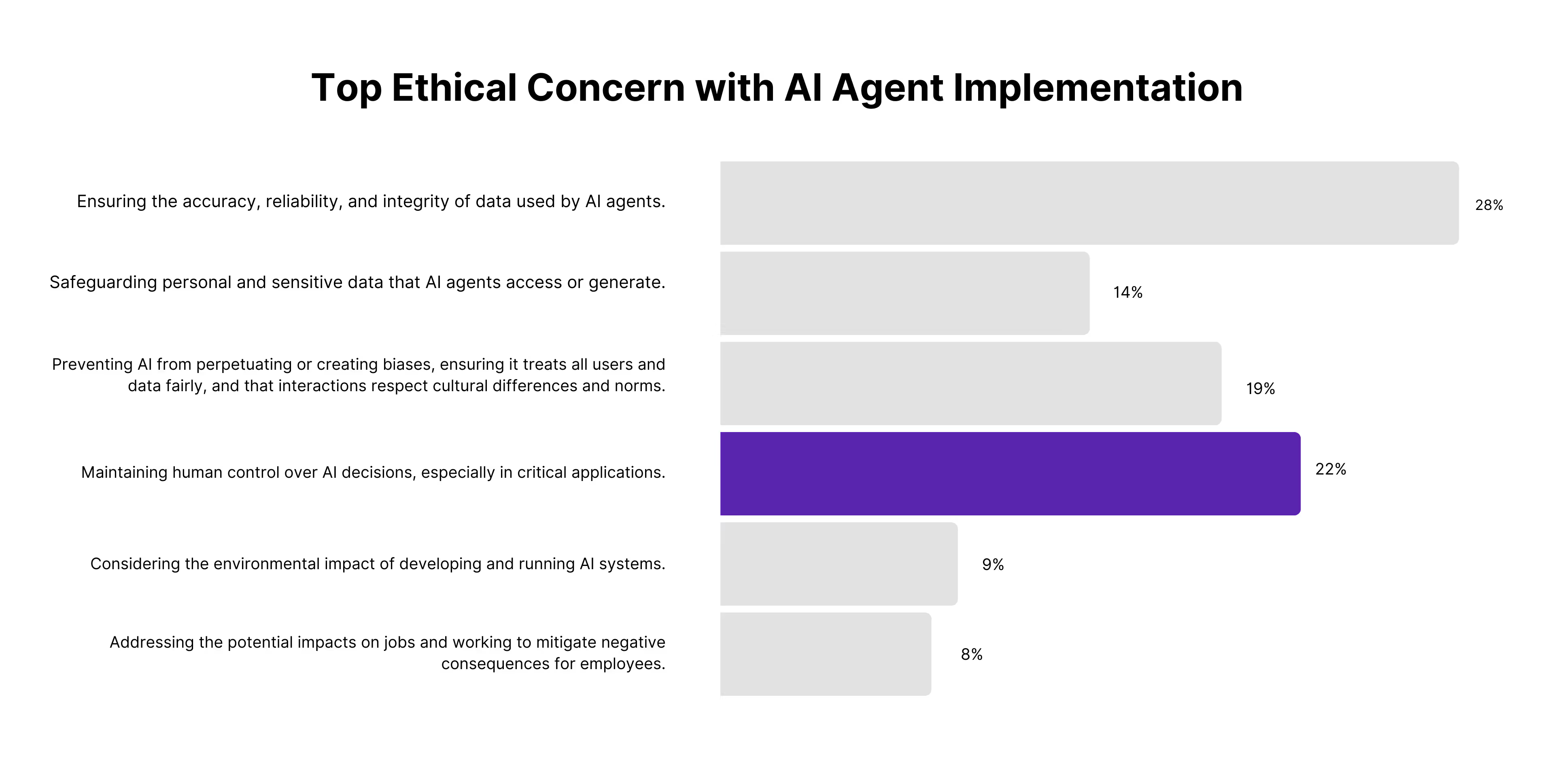
David Magerman, Partner at Differential Ventures, warns against overestimating the capabilities of AI agents, particularly in complex tasks. “While AI agents can handle simpler tasks effectively, they often struggle with more complex workflows without human oversight, leading to irrational outputs that can undermine trust in the technology,” Magerman explains.
One way to solve this is to provide varying levels of human control. Zhang shares that “early enterprise feedback on Personal AI’s solution indicated a desire for different degrees of autonomy. Some users wanted an autopilot mode for less sensitive tasks, while others––those in highly-regulated industries like financial services––needed a copilot mode for more sensitive matters that prevent actions until approved by the user.”
A Roadmap For Startups
According to the data, despite the concerns around trust, 51% of decision-makers are very open to engaging with startups for AI agent solutions, particularly when these startups offer innovative and tailored solutions, as well as speed and agility, that incumbents cannot match.
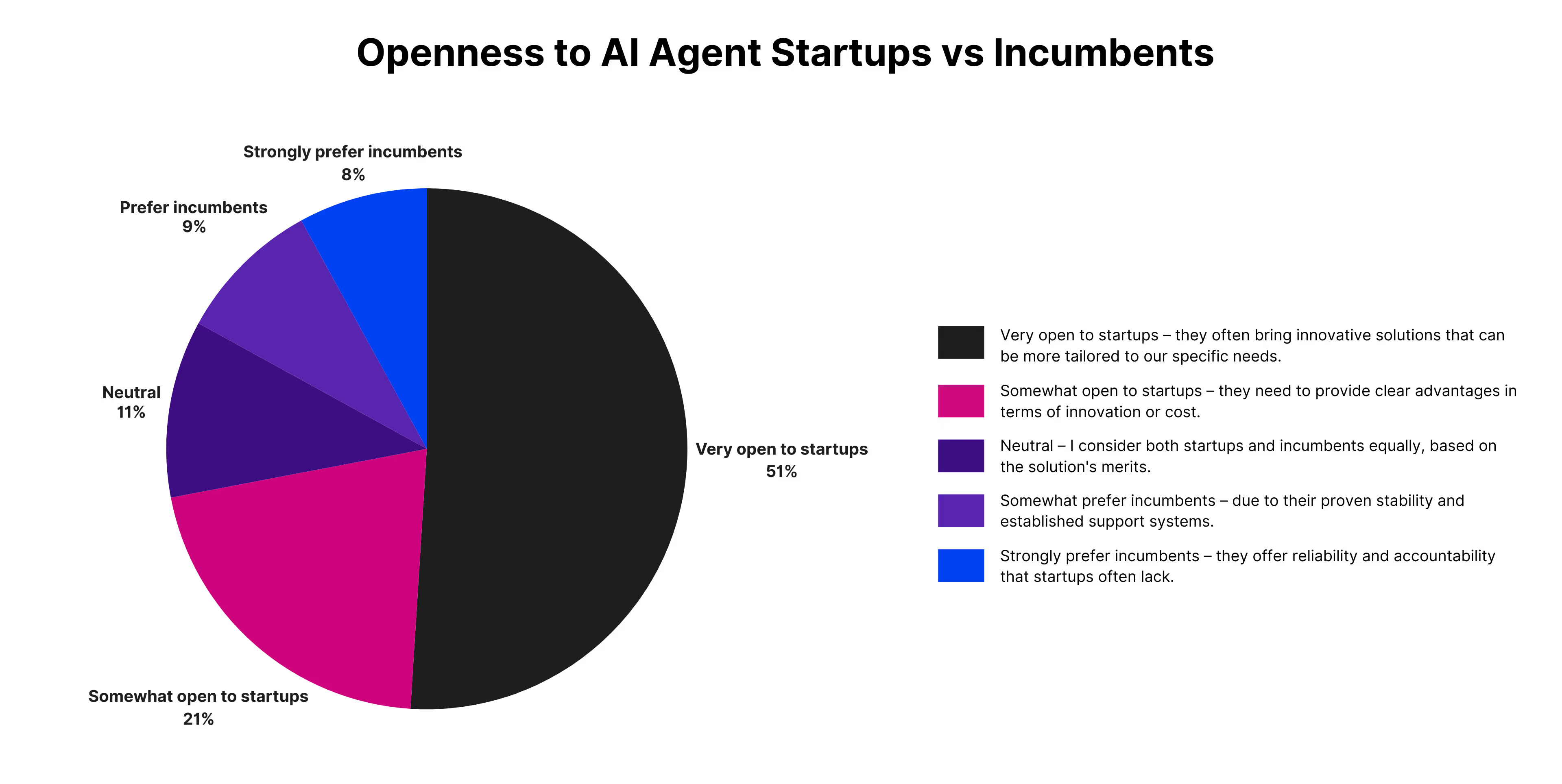
As part of ongoing conversations with founders, enterprises want to understand the solution’s potential to deliver a notable return on investment (ROI). More specifically, 26% of enterprises are primarily looking for ROI in relation to increased operational efficiency, whereby AI agents streamline operations, reduce manual work, and increase throughput. Improved employee productivity, a direct contributor to operational efficiency, was cited by 19% of respondents as a primary expectation for ROI.

"Enterprises are looking for solutions that can deliver immediate value, whether through cost reductions, enhanced performance, or entirely new operational methods,” Midanik explains. “AI agents can supercharge the traditional ‘cheaper, better, faster’ model of software, and startups need to demonstrate how.”
A similar survey insight reveals that IT operations are the top priority for AI agent investment, with 23% of respondents focusing on this area in the next one to two years. The emphasis will be on enhanced system monitoring, predictive analytics, and automated maintenance to reduce downtime and improve operational efficiency.

Below is a roadmap for founders with advice from our contributors on how to show clear, tangible business value and capitalize on the enterprises’ willingness to work with startups at this early stage of AI agents.
1. Educate the Enterprise
Despite the growing interest in agentic AI, many founders report that enterprise buyers often conflate AI agents with simpler, less powerful LLM-based tools like chatbots. This limited understanding is impacting their ability to grasp how these solutions could drive significant operational improvements and strategic growth, and in turn, is affecting the length of a sale.
Tooba Durraze, Ph.D., CEO of Amoeba, emphasizes the importance of education beyond the chatbot paradigm and the real value agents can create for businesses to advance conversations. “What sets agents apart is their potential to not just generate outputs but to evolve toward understanding and applying causal reasoning,” she explains.
As enterprises begin to explore startup solutions, it's crucial for founders to take the time to talk to decision makers across multiple departments outside of IT to understand what they see as the value proposition of AI agents. From here, they can identify knowledge gaps, define things in practical terms, align solutions with business needs and objectives, and ultimately start to build meaningful excitement around the opportunities.
2. Demonstrate Defensibility
To successfully pitch to enterprise customers, founders need to demonstrate clear, tangible ways their AI agent solution is defensible. Sam Strickling, Senior Director of Growth and Innovation at Fortive, explains that “at Fortive, we consider the following when we speak to founders: Do you have unique depth that makes your solution specific enough? Did you bring a proprietary dataset to this? Do you have valuable IP?”.
Startups looking to sell into enterprises should demonstrate uniqueness through proprietary data, intellectual property, or deep industry expertise. These elements set your solution apart, and while patent laws around AI may still be evolving, applying for patents or showcasing something novel can bolster your position.”
3. Showcase Deep Expertise
While horizontal AI agents that address general workflows like customer support have been first to market, Guleri believes that vertical AI agents—designed for specific industries with complex business processes such as financial services, insurance, and pharmaceuticals—will have a stronger competitive moat and drive lasting adoption within enterprises. These industries have the most to gain from specialized AI agents that handle regulatory complexities and unstructured data.
Strickling explains that in industries like supply chain management or semiconductors, offering a one-size-fits-all pitch can undermine credibility. He advises founders to demonstrate deep expertise in a single industry and a passion to drive it forward to resonate deeply with buyers, as it shows a true understanding of the problem you're solving. “It’s clear when someone has built a solution and is now looking for an industry to sell it to, versus someone who has a passion or depth within the industry and has solved a specific problem within it,” Strickling notes.
4. Use Synthetic Data To Prove Potential
When startups are selling into the enterprise, they often need access to proprietary data in order to demonstrate the full potential of their solution. However, enterprises need to see value to provide access to data––and they often need to take strong compliance measures before sharing it. “Herein lies the classic chicken-and-egg problem,” explains Strickling. “This cycle often gets stuck with corporate legal or data privacy teams in the middle."
Strickling advises that startups create mock or synthetic data to demonstrate their agentic solution’s potential: “If you have a sense of the type of data a company might have or be interested in, you can show an example of what the solution could look like in action. This will often get someone, like me, excited enough to champion the idea internally.” This approach is now faster than ever, thanks to the ease of generating synthetic data with AI.
5. Show Ease of Rapid Scalability
Large enterprises want to invest in fewer solutions that can be seamlessly implemented across their organization, ensuring broad applicability and swift, easy deployment.
Strickling explains that Fortive evaluates solutions based on how simple it will be to scale at a company of their size, with 18,000 employees. “If a solution works well for one of our operating companies, I look at how long it will take to achieve adoption across the organization,” Strickling explains. “If the process is too slow, it becomes less appealing.”
Guleri is also bullish on rapid scalability, citing it as one of the key requirements for gaining buy-in across the company. “Enterprises are particularly concerned with the ease of which an AI agent can scale from one department to the entire organization,” explains Guleri.
To build for rapid scalability, startups should design their AI agents with modular architectures that can be easily integrated into existing enterprise systems. This process involves ensuring compatibility with common enterprise software platforms, offering flexible APIs, and creating solutions that are customizable to the specific needs of different departments or functions within an organization.
Demonstrating scalability can be achieved by running smaller pilot programs in targeted business units and showcasing how the solution can be quickly and successfully implemented across other areas of the enterprise. Providing case studies or examples of rapid deployment in similar-sized companies, along with projections of timeframes for full implementation, will further strengthen the pitch.
By emphasizing scalability from the start and showing a clear roadmap for rolling out the solution company-wide, startups can address one of the primary concerns enterprises have when adopting new AI technology.
Predictions for a Not-So-Distant Future
Forum Ventures foresees a profound shift in the way work will be carried out as agentic AI matures. Individuals may soon find themselves primarily communicating with AI agents to describe tasks, review suggested processes, and verify outcomes. This new paradigm could upend how we interact with technology—potentially eliminating the need for websites or apps as we know them. However, realizing this vision will depend on more than just the capabilities of agents; it will require startups to build secure pathways that enable enterprises to provide agents with access to private and sensitive information.
Black echoes this with his vision of a future where AI agents replace traditional user interfaces, taking over tasks that users would typically perform with software. As these agents become more sophisticated, they could manage sensitive activities like 401(k) management or even child care appointments. This shift will significantly alter the knowledge work landscape, enabling AI to handle increasingly complex tasks, thereby boosting productivity. This transition will depend heavily on the agents’ ability to gain trust and authority over time.
The following are predictions from our contributors for how AI agents will evolve to impact how we work, interact with software, and strategically grow businesses.
Specialization and Code Generation Systems
Magerman sees AI agents evolving into highly specialized tools, handling complex tasks like code generation. In his view, these agents will take human instructions and turn them into a series of steps, similar to how a computer program works. He predicts that instead of replacing human intelligence or causing major disruptions, AI agents will serve as an information technology version of robotics, performing specific, repetitive tasks in data-driven environments. This pragmatic approach aligns with his belief that AI agents should be treated as specialized problem solvers, not general-purpose systems.
The Emergence of a Synthetic Workforce
Strickling predicts the rise of a "synthetic workforce" whereby AI agents take on tasks typically handled by junior to mid-level employees. These agents will autonomously learn and execute tasks, showcasing their work and learning process along the way. This capability is expected to mature within the next one to three years, potentially leading to more complex, multi-agent collaborations on larger projects. Strickling also anticipates that AI agents will evolve to not only perform tasks but also manage other agents, acting as supervisors of AI agent teams.
Multi-Agent Networks and Orchestration
Both Zhang and Black foresee the development of multi-agent networks, where different AI agents, each specializing in a particular task, collaborate to achieve common goals that no single agent could accomplish alone. This approach mirrors real-world workflows, where different departments work together to create a final product.
Durraze is excited about the architectural possibilities for multi-agent systems. These could include configurations where agents operate on the same level or where a lead agent coordinates with sub-agents to complete tasks. Another model is a teacher-student architecture, where the student acts as the primary agent while the teacher agent monitors and evaluates outputs to ensure accuracy. “The next step in this evolution,” she adds, “is developing an orchestration layer that can dynamically assemble, configure, and evaluate the multi-agent network.”
From Task-Based to Outcome-Based
Midanik envisions a significant transformation in work structure. Rather than relying on traditional task-based software, future AI agents will offer unified tools that manage comprehensive projects, learn from each experience, deliver outcomes, and achieve goals autonomously. This shift represents a fundamental change in business operations, moving from task-based assistance to providing complete, end-to-end solutions.
True Differentiation will Emerge
Guleri anticipates intense competition in the AI agent space, mainly due to the current hype cycle. However, he believes that true differentiation will emerge in the next 12 to 18 months as companies begin to showcase real value from their AI deployments. As tangible examples of successful AI integration become more common, the initial hype will start to settle, paving the way for more widespread adoption.
An Exciting Path Ahead
As AI agents evolve into sophisticated, autonomous systems, enterprises face unparalleled opportunities to enhance efficiency and drive innovation. However, adoption depends heavily on overcoming key barriers, particularly trust. To gain traction, founders must not only deliver measurable value but also ensure transparency, reliability, and a strong commitment to data privacy and security.
Startups should focus on demonstrating ROI through pilot projects, leveraging synthetic data where necessary, and emphasizing scalability. Enterprises, meanwhile, should take a gradual approach to adoption—starting with small implementations before scaling—while maintaining human oversight to ensure AI agents align with their specific needs, company values, and regulatory requirements.
The future of agentic AI promises a shift toward goal-oriented systems that will transform business operations and offer new possibilities across industries. Building trust, delivering impact, and fostering human-AI collaboration will be crucial to achieving widespread adoption.
Latest Playbooks
.avif)